This article is part of the MITB Thought Leadership Series published by the SMU School of Information Systems’ Master of IT in Business programme.
By Seema Chokshi, Lecturer, SMU School of Information Systems
What is big data? The intuitive meaning of the phrase ‘big data’ might be “data that is huge in quantity”. But is that interpretation enough? Data of this type has existed for as long as humans have made records of their work. Some of the earliest writings, such as cuneiform, contain vast amounts of data covering areas as diverse as law, mapping and mathematical equations. In the modern era, corporations were using millions of paper records decades before the invention of large-scale digital storage in the 1990s. So what is new?
While the definition of data itself has not changed—data will always form the building blocks of information—‘big’ refers to the ‘volume’, ‘variety’ and ‘velocity’ of that data. To put it in simpler terms: Big data refers to data that is massive in volume, changes very rapidly, and consists of many diverse types.
The surge in this type of data took place in the mid-2000s, when much larger percentages of data began to be created by users interacting with applications on the Internet. Unlike the pre-social media era when companies were the main creators of data—on customers, for their portfolios—suddenly, all sorts of new sources appeared or scaled-up: data from people writing reviews; commenting on Facebook; posting news to Twitter, and so on.
To add to this, machines began creating their own data in much larger quantities. Automated sources such as sensors, satellites and CCTV systems began generating and storing data every micro-second of every day, over periods of months and years.
Why the interest in big data from industry?
With all these ones and zeros being created and stored, businesses identified early on, the opportunities that extracting insight might bring. But more often than not, the processing speeds of the technology required to do this was insufficient.
Then Apache Hadoop appeared. This open source tool developed by the U.S. non-profit Apache Software Foundation group was based on an innovative way to reduce the time taken to process data pools using multi-core processors in parallel. Many banks and corporations were quick to use it, helping them run algorithms for information processing in a fraction of the time taken before. When software vendors like Cloudera were formed to offer enterprise-class deployments of the technology, adoption widened to many more large organisations around the world.
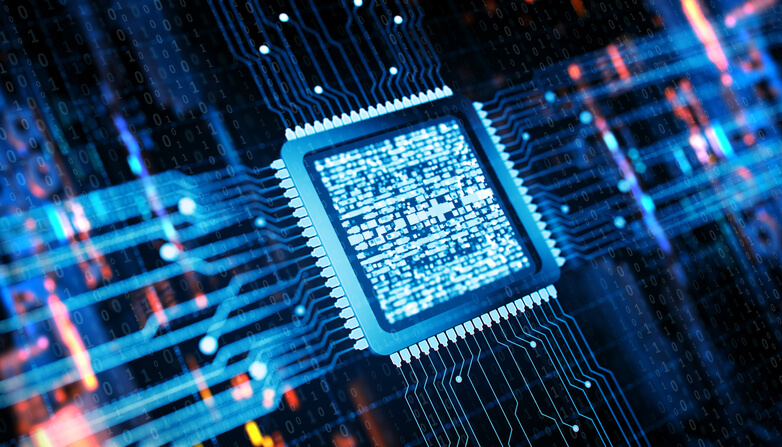
What did this mean for consumer businesses in general?
Companies could now get creative. They used data science algorithms to implement customer strategies that were hitherto impossible to execute. Whether it was acquisition, customer management, customer service—indeed any aspect of customer lifecycle—the ability to make real-time business decisions meant a big win for the organisation. An example of this rapid evolution lies in customer recommendations.
Learning from consumer behaviour
Recommendation systems are all around us today. They are used while making a purchase on an ecommerce site like Amazon or Lazada; when choosing a film to watch on Netflix; when looking for a job on LinkedIn—even while listening to music on a web-based radio channel. The work on this field started in the 1990s, but it gained real momentum more recently as the technological advances already discussed began to gather steam.
Every time a customer buys one of the more than 400 million products that are listed on Amazon.com, the data is stored, updated, and a new product is recommended to the buyer based on their most recent purchase. The offer is created by multiple recommendation algorithms—or collaborative filtering algorithms to give them their technical term—which harnesses the purchase decisions made by millions of other users who have previously bought from the platform.
“The use of big data comes with big responsibility.”
The challenges ahead
An article written for Harper’s magazine in 1989 by the well-known author Erik Larson included these lines: “The keepers of big data say they do it for the consumer’s benefit. But data have a way of being used for purposes other than originally intended.” He was referring to the junk email that he received, but the problem is today much, much wider.
The use of big data comes with big responsibility. Issues of consumer data collection and privacy violation – distilled in the phrase ‘surveillance marketing’—have been highlighted repeatedly as scandals involving both the highest profile and the most opaque companies have erupted. Still, radical personalisation is still seen by many as the positive aspect of the technology that balances these concerns.
If a healthcare company is alert to unusual data on a particular health metric, it can make an intervention with preventive care. If a film company understands a viewer’s interests, it can suggest titles a customer really wants to watch. And if a credit card company has a complete credit profile of an individual, it can offer credit with reduced risk of default.
For a data scientist, it is a dream to process all this data and to apply machine learning algorithms to it in order better to understand—and shape—the world.
Seema Chokshi is currently a Lecturer of Information Systems and programme at Singapore Management University (SMU) and programme director of the SMU Undergraduate Second Major in Analytics. Seema has over 13 years of professional experience as an educator and as an industry consultant. View her profile here.