Breaking Analysis Paralysis with the Help of Artificial Intelligence
Recommendations are useful for helping us find new and exciting content and products. As part of his research, PhD in Computer Science graduate Truong Quoc Tuan developed algorithms and intelligent systems that can extract information about a user's sentiment or preference for various products. Together with Associate Professor Hady Lauw, they discuss how these algorithms can be used efficiently and safely in the first episode of podcast series Theory of Curiosity.
Is there anything better than finding an outfit that fits like a glove, or a gadget that changes your life? Or finding a new book, movie, or TV show to binge-watch without having to comb through reviews and make comparisons across multiple platforms?
Recommendations are useful in helping us find new and exciting content and products. But what if recommendations can be made better? What if we can utilise artificial intelligence-assisted suggestions to help us find products that are tailored specifically for us, based on our user behaviour? What if we can spend less time browsing through irrelevant content, and more time enjoying the things we love?
In the first episode of Theory of Curiosity, a podcast series where listeners get inside the inquisitive minds of SMU’s brightest researchers, Associate Professor of Computer Science Hady Lauw chats with PhD in Computer Science graduate, Truong Quoc Tuan, about how technology can customise online experiences.
Leveraging artificial intelligence and machine learning
Assoc Prof Hady believes the key lies in leveraging artificial intelligence and machine learning to uncover user preferences from data. As part of his research, Tuan had developed algorithms and intelligent systems that could extract information about a user’s sentiment or preference for various products.
“If you look at the activities you do on the web, you need recommendations most of the time,” explains Tuan.
“Recommender systems play a key role in activities like watching movies, online shopping, listening to music, and interacting on social networks. Different users have their preferences and are unique in the sense that we need to recommend items or products that suit their tastes.”
Today, a plethora of choices besiege consumers. With over 15,000 titles on Netflix and 700 listings for “chocolate milk” on online grocer RedMart alone, it’s no wonder that the digital generation suffers from analysis paralysis.
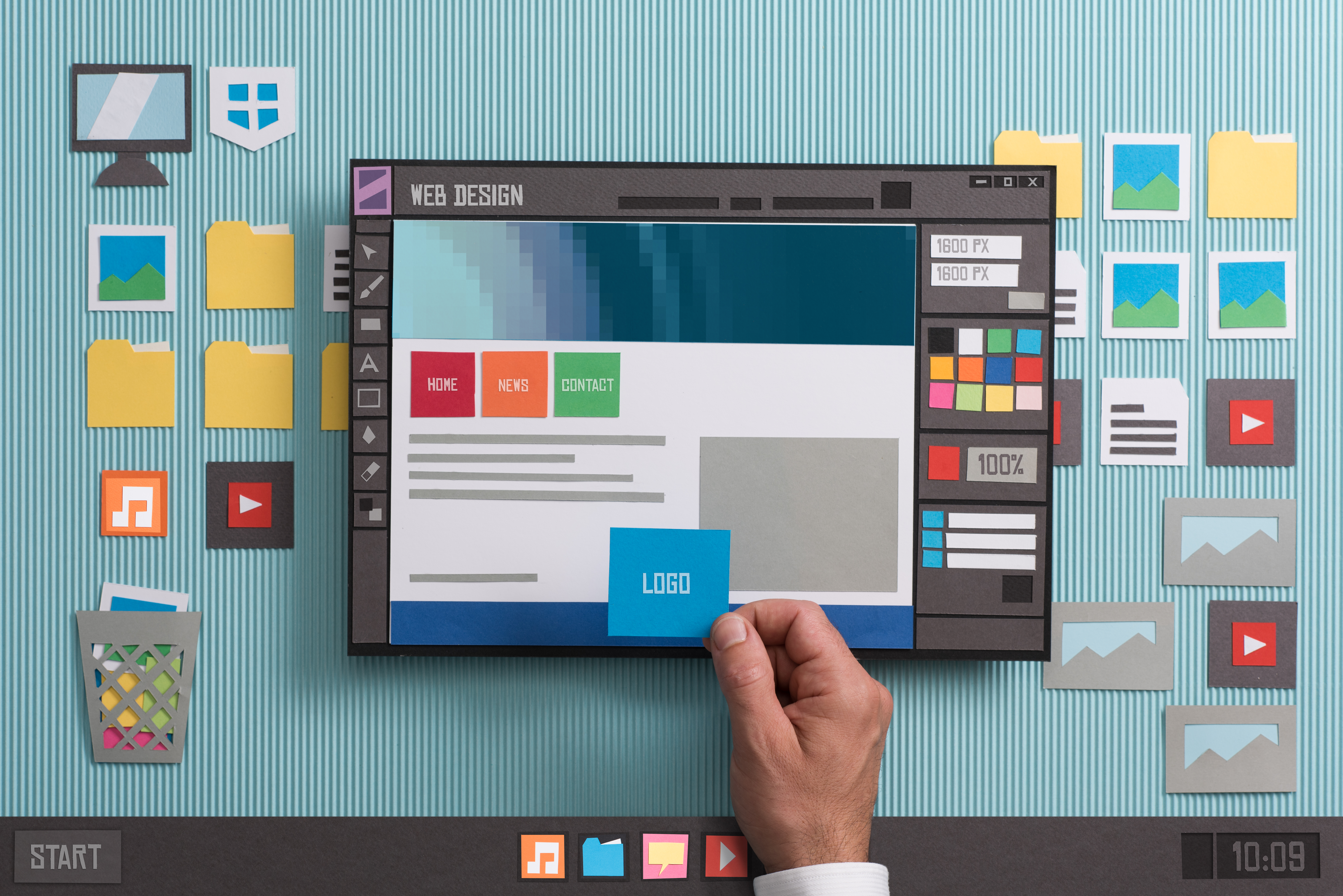
“If you look at the activities you do on the web, you need recommendations most of the time.”
Truong Quoc Tuan
PhD in Computer Science graduate
However, making product recommendations goes beyond a marketer pitching their brand’s newest launch. Computer scientists have developed sophisticated programmes to analyse enormous volumes of data to aid users’ decisions on online platforms and ensure the picks meet their needs, says Tuan, whose research interests include user profiling and personalisation.
Such recommender systems tap on various forms of data to customise recommendations. For example, a user’s interactions with a product, such as clicking on a listing, reading about the item, or purchasing it in the past, play a crucial role in machine-assisted suggestions. Other kinds of data, such as user reviews or the descriptive content of products, also contribute to a match.
As Tuan adds: “Using the interactive data between a user and items, we tried to extract similarities or patterns and recommend items that similar users might like, even if the user has not been exposed to the items before.”
One algorithm fits all
The beauty of tech-enabled recommendation systems is that the algorithm can be applied across different products, industries, platforms and markets. Rather than building a system from scratch to make movie recommendations and another for fast-selling fashion, businesses may employ generalised formulations into their systems.
“A large portion of my dissertation focused on designing efficient algorithms that rely on primary interactions between users and items, which are limited as we have a large number of items and most of the time, those items are not exposed to the users,” elaborates Tuan, whose dissertation is on Modelling Sentiments and Preferences from Multimodal Data.
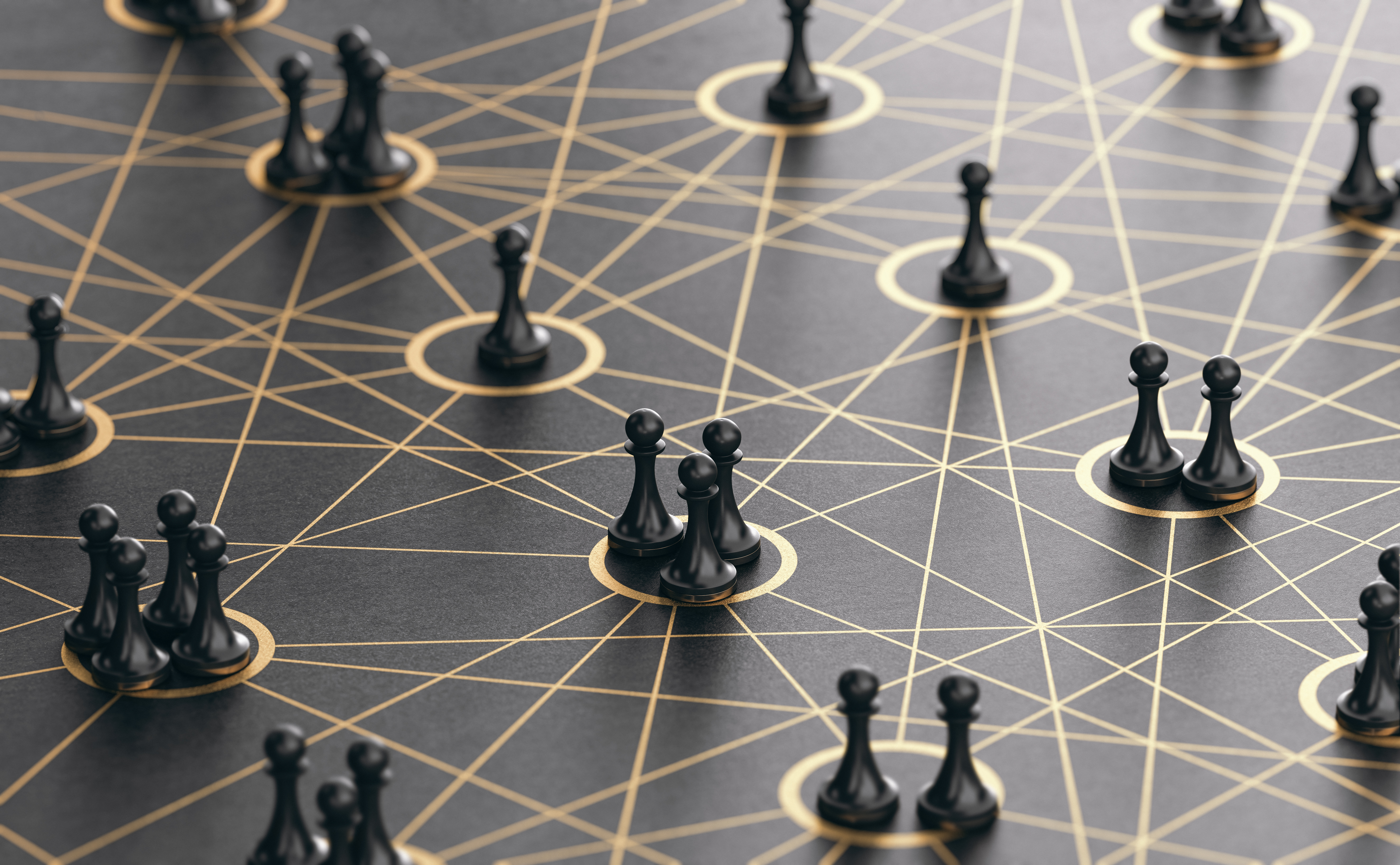
Based on a user’s past interactions, the recommendation system can suggest the kinds of items a customer prefers.
“We need to also look at other forms of interactions, or other forms of which we call ‘modalities’ that appear in those data, like text or images of the items, or the social connection of the users. The additional information helps bootstrap the primary interactions to provide more accurate recommendations to users.”
Images, for example, play a significant role in shopping for fashion items. Based on a user’s past interactions, the recommendation system can suggest the kinds of items a customer prefers. On the other hand, book recommendations can be based on the item’s textual content, such as its title and abstract. Interestingly, these systems don’t just introduce selections that are already popular. They expose users to new items that would not have otherwise been on their radar.
Data privacy
As much as recommendation systems make the lives of digital citizens that much easier, the issue of privacy often arises in the collection of data.
“Personally, there are certain contexts in which I don’t mind showing some of this data,” says Assoc Prof Hady.
“If it relates to the kind of news or books I’m reading, that’s perfectly fine, because it will help the system recommend better news, better books, and so on. But there could be some other domains such as medical services, where I might be a bit more careful about having that sort of data shared, even if it means I might be recommended a better doctor.”
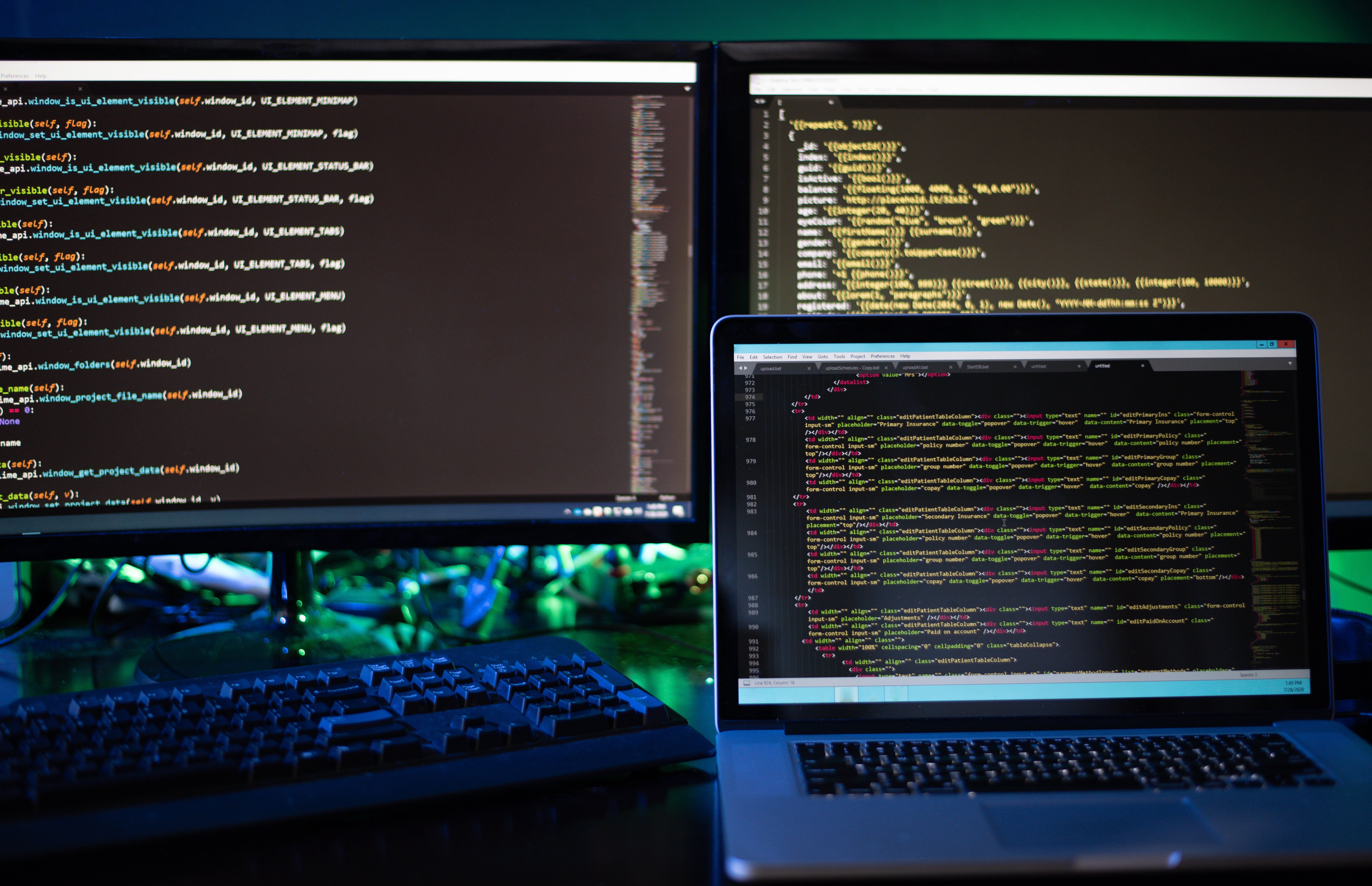
“I believe a PhD in computer science opens up many doors upon graduation.”
Truong Quoc Tuan
PhD in Computer Science graduate
Ultimately, Assoc Prof Hady believes that education is essential to help users understand that their preferences are being tracked, and that they have some means for controlling the level in which their data is shared. Moreover, he notes that technological breakthroughs arising from research help redefine existing industries and how we work globally.
“Most people think of PhD training as a closed door, you finish your PhD and then you have to become a professor,” relates Tuan.
“But I believe a PhD in computer science opens up many doors upon graduation. The skills we gain help us advance existing knowledge, approach new problems more systematically, and make ideas a reality.”
"Recommender Systems: The Future of Personalised Online Experiences” is the first episode of Theory of Curiosity – a podcast series dedicated to showcasing the findings of SMU’s postgraduate research professors and students on topics in digital transformation, growth in Asia and sustainable living. Listen and subscribe to the full podcast series here.